Prof. Shiwen Mao
Auburn University, Auburn, Alabama, US
Title: Indoor Localization with Deep Gaussian Process and Uncertainty Maps
Bio:
Shiwen Mao is a professor and Earle C. Williams Eminent Scholar, and Director of the Wireless Engineering Research and Education Center (WEREC) at Auburn University, Auburn, AL, USA. His research interest includes wireless networks, multimedia communications, and smart grid. He is a Distinguished Lecturer of IEEE Communications Society (ComSoc) and the IEEE Council of RFID. He is the Editor-in-Chief of IEEE Transactions on Cognitive Communications and Networking and on the Editorial Board of several other IEEE and ACM journals. He received the IEEE ComSoc TC-CSR Distinguished Technical Achievement Award in 2019 and NSF CAREER Award in 2010. He is a co-recipient of the 2021 Best Paper Award of Elsevier/KeAi Digital Communications and Networks Journal, the 2021 IEEE Internet of Things Journal Best Paper Award, the 2021 IEEE Communications Society Outstanding Paper Award, the IEEE Vehicular Technology Society 2020 Jack Neubauer Memorial Award, the 2004 IEEE Communications Society Leonard G. Abraham Prize in the Field of Communications Systems, and several conference and ComSoc best paper/demo awards. He is a Fellow of the IEEE.
Abstract:
With the increasing demand for location-based service, WiFi-based localization has become one of the most popular methods due to the wide deployment of WiFi and its relatively low cost. In this talk, we present a deep Gaussian process based indoor radio map construction and location estimation system. Received signal strength (RSS) samples, as well earth magnetic field readings, are used to generate accurate and fine-grained radio maps with confidence intervals using deep Gaussian process, while the model parameters are optimized with an offline Bayesian training method. Utilizing the maps, an LSTM based location prediction model is pre-trained with the artificial trajectory data and then fine-tuned with the signal measurements collected by the mobile device to be localized. Our extensive experiments demonstrate the excellent performance of the proposed system.
Prof. Yan Zhang
University of Oslo, Norway
Bio:
Yan Zhang is currently a Full Professor with the Department of Informatics, University of Oslo, Norway. His research interests include next-generation wireless networks leading to 6G, green and secure cyber-physical systems. Dr. Zhang is an Editor for several IEEE transactions/magazine, including IEEE Network Magazine, IEEE Transactions on Green Communications and Networking, IEEE Transactions on Network Science and Engineering, IEEE Transactions on Sustainable Computing, IEEE Transactions on Vehicular Technology, IEEE Transactions on Industrial Informatics, IEEE Internet of Things Journal, IEEE Systems Journal, and IEEE Vehicular Technology Magazine. He is a program/symposium chair in a number of conferences, including IEEE IWQoS 2022, IEEE ICC 2021, IEEE SmartGridComm 2021. He is the Chair of IEEE Communications Society Technical Committee on Green Communications and Computing (TCGCC). He is an IEEE Communications Society Distinguished Lecturer and IEEE Vehicular Technology Society Distinguished Speaker. He was an IEEE Vehicular Technology Society Distinguished Lecturer during 2016-2020. Since 2018, Prof. Zhang was a recipient of the global “Highly Cited Researcher” Award (Web of Science top 1% most cited worldwide) for four years. He is Fellow of IEEE, Fellow of IET, elected member of Academia Europaea (MAE), elected member of the Royal Norwegian Society of Sciences and Letters (DKNVS), and elected member of Norwegian Academy of Technological Sciences (NTVA).
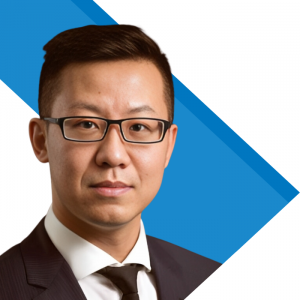
Prof. Miao Pan
University of Houston, Texas, US
Title: To Talk or to Work: Efficient Federated Learning over Mobile Devices
Bio:
Dr. Miao Pan is an Associate Professor in the Department of Electrical and Computer Engineering at University of Houston. He was a recipient of NSF CAREER Award in 2014. Dr. Pan received his Ph.D. degree in Electrical and Computer Engineering from University of Florida in August 2012. Dr. Pan’s research interests include wireless/AI for AI/wireless, deep learning privacy, wireless networks, underwater communications and networking, and cybersecurity. He has published more than 90 papers in prestigious journals including IEEE/ACM Transactions on Networking, IEEE Journal on Selected Areas in Communications, IEEE Transactions on Mobile Computing, and IEEE Transactions on Smart Grid, and around 130 papers in top conferences such as IEEE INFOCOM, ACM MobiSys, IEEE ICDM, AAAI, ACM CIKM, IEEE BigData, ICDCS, and IEEE IPDPS. His work won IEEE TCGCC (Technical Committee on Green Communications and Computing) Best Conference Paper Awards 2019, and Best Paper Awards in ICC 2019, VTC 2018, Globecom 2017 and Globecom 2015, respectively. Dr. Pan serves as a Technical Reviewer for many international journals and conferences. He has also been serving as a Technical Organizing Committee for several conferences such as TPC Co-Chair for Mobiquitous 2019, ACM WUWNet 2019, and Technical Program Committee member of several top international conferences, e.g., IJCAI, IEEE INFOCOM, MobiHoc, ICDCS, etc. Dr. Pan is an Editor for IEEE Open Journal of Vehicular Technology and an Associate Editor for IEEE Internet of Things (IoT) Journal (Area 5: Artificial Intelligence for IoT), and used to be an Associate Editor for IEEE Internet of Things (IoT) Journal (Area 4: Services, Applications, and Other Topics for IoT) from 2015 to 2018. Dr. Pan is a member of AAAI, a member of ACM, and a senior member of IEEE and IEEE Communications Society.
Abstract:
In the era of AI and mobile Internet, federated learning (FL) over mobile devices, one of the most anticipated technologies, has fostered numerous intriguing applications and services, while many of them are energy-hungry and delay-sensitive. In this talk, I would like to discuss our proposed energy/delay efficient FL designs over mobile devices. Different from most existing communication efficient FL designs, which regard wireless communications as the bottleneck, we have an interesting observation that under many typical FL settings, the local computing energy/delay is comparable to the communication energy/delay during the FL training process, given the development of high-speed wireless transmission techniques (e.g., 4G LTE, 5G, Wi-Fi 5 or Wi-Fi 6). Thus, the total energy/delay should be computing energy/delay + communication energy/delay over training rounds in FL. To effectively reduce the overall energy/delay of FL over mobile devices, simply reducing local computing/communication energy/delay independently is not enough, and the energy/delay trade-off between “working” (i.e., local computing) and “talking” (i.e., wireless communications) has to be considered. To study this trade-off, we have initially modeled and empirically studied the impacts of advanced local computing control (e.g., DVFS, local iteration number), compression (e.g., gradient quantization, gradient sparsification) and wireless transmission (e.g., transmission rates) strategies on computing and communication energy/delay, formulated the problem of efficient FL over mobile devices, and provided feasible solutions. We have also set up a testbed of FL over mobile devices with Lambda server, Nvidia TX2s/Xaviers and NI USRPs to validate our analysis, and shown promising experimental results.
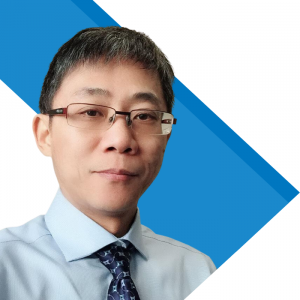
Prof. Jun Cai
Concordia University, Canada
Bio:
Jun Cai received the Ph.D. degree from the University of Waterloo, ON, Canada, in 2004. From June 2004 to April 2006, he was with McMaster University, Canada, as a Natural Sciences and Engineering Research Council of Canada (NSERC) Postdoctoral Fellow. From July 2006 to December 2018, he has been with the Department of Electrical and Computer Engineering, University of Manitoba, Canada, where he was a full Professor and the NSERC Industrial Research Chair. Since January 2019, he has joined the Department of Electrical and Computer Engineering, Concordia University, Canada, as a full Professor and the PERFORM Research Chair. His current research interests include edge/fog computing, wireless body area networks, ehealth, digital twin, machine learning, radio resource management, and performance analysis. Dr. Cai served as the General Chair of IEEE BSC 2023; Technical Program Committee (TPC) Co-Chair for IEEE GreenCom 2018; Track/Symposium TPC Co-Chair for the IEEE VTC-Fall 2019, IEEE CCECE 2017, IEEE VTC-Fall 2012, IEEE Globecom 2010, and IWCMC 2008. He also served on the editorial board of IEEE Internet of Things Journal, IEEE Wireless Communications Magazine, IEEE Systems Journal, IEEE Access, and Wireless Communications and Mobile Computing.
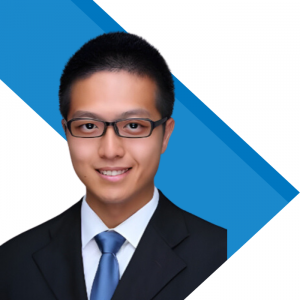
Prof. Ximeng Liu
Fuzhou University, China
Title: Secure Computation: Current and Future Trends
Bio:
Ximeng Liu (S’ 13-M’ 16-SM’ 21) received the B.Sc. degree in electronic engineering from Xidian University, Xi’an, China, in 2010 and the Ph.D. degree in Cryptography from Xidian University, China, in 2015. Now he is the full professor in the College of Mathematics and Computer Science, Fuzhou University. He was a research fellow at the School of Information System, Singapore Management University, Singapore. He has published more than 250 papers on the topics of cloud security and big data security including papers in IEEE TC, IEEE TIFS, IEEE TDSC, IEEE TPDS, IEEE TKDE, IEEE IoT Journal, and so on. He awards “Minjiang Scholars” Distinguished Professor, “Qishan Scholars” in Fuzhou University, and ACM SIGSAC China Rising Star Award (2018). His research interests include cloud security, applied cryptography and big data security.
Abstract:
Cloud computing, an integral part of computer infrastructure, is the delivery of computing services, including storage, databases, servers, networking, software, analytics. Data outsource computation, as one of the most important applications of the cloud, has become more and more attractive. Despite the advantages provided by outsourced data analytics, the individual clients are suspected to outsource their own data to the cloud without any security and privacy guarantee. In this talk, Dr. Liu will offer a new secure computation framework which balances the efficiency and security. The new framework can be applied to construct secure data mining analytic for real-world application, such as e-healthcare, remote authentication.
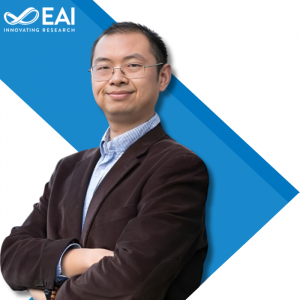
Wenjia Li, Ph.D.
Associate Professor of Computer Science
New York Institute of Technology
New York City, NY
USA
Title: Toward Secure and Trustworthy Vehicle Sensing Using Blockchain Technology
Bio:
Dr. Wenjia Li is an Associate Professor at the CoECS at NYIT. His main research focuses are on cybersecurity, networking, and mobile computing. He studies trust management policies in wireless networks; cyber-physical systems (such as intelligent transportation systems); the internet of things; and Android mobile devices. Dr. Li’s research has been supported by the National Institute of Health (NIH), US Department of Transportation (USDOT). He has published over 100 papers in various international journals and conferences. Dr. Li was a recipient of the 2019 IEEE Region 1 Technological Innovation (Academic) Award. He also received the NYIT Presidential Award for Student Engagement in Research, Scholarship, or Creative Activities in 2020. Dr. Li obtained the PhD degree in Computer Science from University of Maryland Baltimore County (UMBC) in 2011. He has served on the organizing and program committee for various international conferences, such as IEEE INFOCOM, IEEE GLOBECOM, IEEE ICC, IEEE WCNC, IEEE IPCCC, IEEE VTC, IEEE MDM, and so on. In addition, Li has reviewed papers for many top-tier peer-reviewed journals. He is a senior member of IEEE and a member of ACM.
Abstract:
In recent years, connected vehicles have gradually stepped into our daily lives, and they generally rely on vehicular networks to generate and share different types of sensing data to improve overall travel safety and efficiency. However, due to the open nature of vehicular networks, these sensing data could be erroneous, which may be caused by various reasons, ranging from an onboard device (OBD) sensor malfunctioning and reporting incorrect reading to the sensing data being tampered by a malicious vehicle. This talk will address the research question of how to enhance security and trust in vehicular networks and discuss how blockchain technology helps achieve that. We will first introduce the research background regarding security and trust management, and then provide a general overview of the blockchain-enabled trust management approach for vehicular networks.
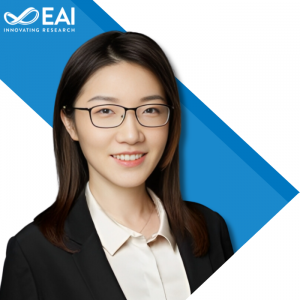
Xiaoqi Qin
Beijing University of Posts and Telecommunications
Title: Jane machine communication and Networked cognitive technology
Bio:
Dr. Xiaoqi Qin is currently an Associate Professor at Beijing University of Posts and Telecommunications. She is a member of State Key Laboratory of Networking and Switching Technology, as well as an Outstanding Beijing Undergraduate Education and Teaching Team. Dr. Qin received her B.S., M.S., and Ph.D. degrees in Electrical and Computer Engineering from Virginia Tech. Her research focuses on exploring performance limits of next-generation wireless networks,and developing innovative solutions for intelligent machine-type communications. She has published over 60 papers in top journals and conference, including Engineering, IEEE JSAC/TWC/TMC, ACM Mobisys, and has authored one book. She has undertaken several national projects from National Natural Science Foundation and National Key Research and Development Program. She has been awarded “Distinguished Young Investigator of China Frontier of Engineering”, 1st Prize of Beijing Higher Education Teaching Achievement Award, and 1st Prize of Tech. Invention Award by China Institute of Communications. Moreover, she has received the support of China Association for Science and Technology Young Talent Project. Dr. Qin also serves as Young Expert of China Intelligent Transportation Systems Association,Deputy Director of ZTE Industry-University-Research Cooperation Committee,and Associate Editor for several journals.
Abstract:
Traditionally, wireless connectivity has been regarded as a “pipe” delivering data to humans, treating each data bit independently and equally. The context-dependent effectiveness of transmitted data is ignored and left to human perception. Therefore, the design philosophy of wireless communication system mainly focuses on throughput maximization. However, as the proliferation of real-time networked control applications, massive intelligent machines are now connected to accomplish tasks, such as industrial automation. This paradigm shift involves information continuously flowing around “sense-transmit-decision” cycles. Due to the dynamic environment, the effective information contained in status updates collected by sensors degrades over time, and outdated information is deleterious to decision performance. Therefore, the decision model should be updated based on the continuously uploaded status data flow. This lecture primarily focuses on introducing task-oriented communication framework for networked intelligent machines,which will be of significant importance in real-time remote control systems.
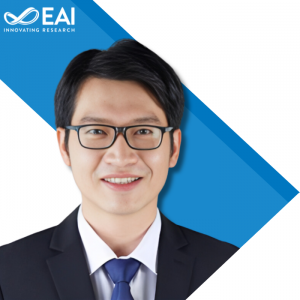
Joey Tianyi Zhou (周天异)
Senior Scientist /Adjunct Associate Professor Centre for Frontier AI Research, A STAR (Adjunct faculty at National University of Singapore)
Title: Can model compression really save energy?
Bio:
Joey Tianyi Zhou is currently a senior scientist, Investigator and group manager with A*STAR Centre for Frontier AI Research (CFAR), Singapore. He is also holding an adjunct faculty position at National University of Singapore (NUS). Before working at IHPC, he was a senior research engineer with SONY US Research Center in San Jose, USA. Dr. Zhou received a Ph.D. degree in computer science from Nanyang Technological University (NTU), Singapore. His current interests mainly focus on improving the efficiency and robustness of machine learning algorithms. %On these areas, he has co/authored more than 100 papers and received the Best Poster Award Honorable Mention at Asian Conference on Machine Learning (ACML’12), Best Student Paper Nomination at European Conference on Computer Vision (ECCV’16), Best Paper Award at International Joint Conference on Artificial Intelligence (IJCAI’16) workshop on BeyondLabeler, IJCAI’19 workshop on BOOM, and Best Poster Award and runner-up prize at International Conference on Computer Vision (ICCV’19) on HANDS workshop and its competition, respectively.
Dr. Zhou organized ICDCS’ 20-21 workshop on Efficient AI meets Edge Computing, ACML’16 workshop on Learning on Big Data workshop and IJCAI’19 workshop on Multi-output Learning; is serving as an Associate Editor for IEEE Transactions on Emerging Topics in Computational Intelligence (TETCI) and IEEE Access, IET Image Processing, and TPC Chair in Mobimedia 2020; and received NeurIPS Best Reviewer Award in 2017.
Abstract:
Edge devices demand low energy consumption, cost and small form factor. To efficiently deploy convolutional neural network (CNN) models on edge device, energy-aware model compression becomes extremely important. However, existing work did not study this problem well because the lack of considering the diversity of dataflow types in hardware architectures. In this talk, I will introduce our recent work named EDCompress, an Energy-aware model compression method for various Dataflows. It can effectively reduce the energy consumption of various edge devices, with different dataflow types. Considering the very nature of model compression procedures, we recast the optimization process to a multi-step problem, and solve it by reinforcement learning algorithms. Experiments show that EDCompress could improve 20X, 17X, 37X energy efficiency in VGG-16, MobileNet, LeNet-5 networks, respectively, with negligible loss of accuracy. EDCompress could also find the optimal dataflow type for specific neural networks in terms of energy consumption, which can guide the deployment of CNN models on hardware systems.
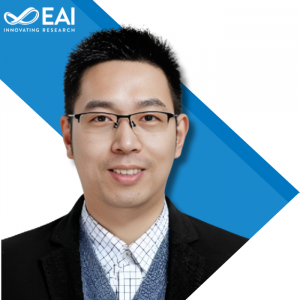
Prof. Weidang Lu
Zhejiang University of Technology
Title: Secure Communication in UAV-assisted Mobile Edge Computing Networks
Bio:
Dr. Weidang Lu received the Ph.D. degree in Information and Communication Engineering from Harbin Institute of Technology in 2012. He was a visiting scholar with the Nanyang Technology University, Singapore, The Chinese University of Hong Kong, China and Southern University of Science and Technology, China. He is currently a Professor with the College of Information Engineering, Zhejiang University of Technology, Hangzhou, China. His current research interests include UAV communication, intelligent communication, secure communication and mobile edge computing. His works received several awards, including Zhejiang natural science award, Jiangxi natural science award, best paper awards of WiSATS 2019 and AICON 2021.
Abstract:
Equipped with mobile edge computing (MEC) servers on UAVs, it can not only save the cost of installing physical infrastructure on the ground and overcome the limitations and shortcomings of ground edge computing, but also achieve fast and timely processing of computing tasks for terminal devices, improve user service quality, and reduce consumption of wireless resources. However, due to the broadcast characteristics of wireless communications, it is easy for malicious users to eavesdrop on the data offloaded from terminal devices to UAVs, which poses a great risk to the secure transmission of data. In this talk, I would like to discuss the data secure transmission issue in UAV-assisted MEC systems and provide a security guarantee mechanism for data offloading from the perspective of physical layer security. Through exploring the inherent connection between data offloading and data secure transmission, to bring the advantages of fast task processing brought by UAV-assisted MEC, while satisfying data security.
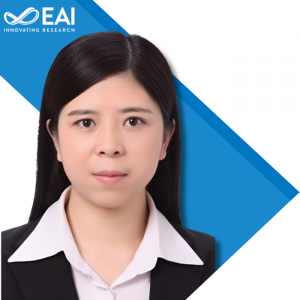
Zhihui Liu
State Key Laboratory of Space-Ground Integrated Information Technology
Beijing Institute of Satellite Information Engineering
Title: Space-Ground Converged Intelligent Networking Technology
Bio:
Zhihui Liu was born in 1989. She received her Ph.D. degree at the School of Information and Communication Engineering from Beijing University of Posts and Telecommunications (BUPT), Beijing, China, in signal and information processing. She is currently a senior engineer in State Key Laboratory of Space-Ground Integrated Information Technology, Beijing Institute of Satellite Information Engineering. Her research interests include space communication and networks, resource management and control for heterogeneous networks, and network resource sensing.
Abstract:
The technology of space-ground converged networking is the key to realize internetworking and information transmission, and becoming increasingly important, as an important part of future network development. The space-ground converged networking involves multiple elements, and thus, there is an urgent need to setting up research on related technologies systematically. In this speech, key issues in three aspects, i.e., network autonomous management and control, fine-grained sensing of network resources, and intelligent managing and scheduling of multi-dimensional resources, are introduced with some solution ideas. It is ended with an outlook on application prospects.