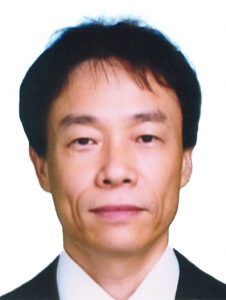
Prof. Nei Kato
Dean with Graduate School of Information Sciences, Tohoku University, Japan
Title: Multi-AP Coordination Approaches over Emerging WLANs: Future Directions and Open Challenges
Bio:
Nei Kato is a full professor and the Dean with Graduate School of Information Sciences, Tohoku University. He has researched on computer networking, wireless mobile communications, satellite communications, ad hoc & sensor & mesh networks, UAV networks, AI, IoT, and Big Data. He is the Editor-in-Chief of IEEE Internet of Things Journal, the fellow committee chair of IEEE VTS. He is a Fellow of the Engineering Academy of Japan, a Fellow of IEEE, and a Fellow of IEICE.
Abstract:
The 802.11 IEEE standard aims to update current Wireless Local Area Network (WLAN) standards to meet the high demands of future applications, such as 8K videos, augmented/virtual reality (AR/VR), the Internet of Things, telesurgery, and more. Two of the latest developments in WLAN technologies are IEEE 802.11be and 802.11ay, also known
as Wi-Fi 7 and WiGig, respectively. These standards aim to provide Extremely High Throughput (EHT) and lower latencies. IEEE 802.11be includes new features such as 320 MHz bandwidth, multi-link operation, Multi-user Multi-Input Multi-Output (MIMO), orthogonal frequency-division multiple access, and Multiple-Access Point (multi-AP) cooperation (MAP-Co) to achieve EHT. With the increase in the number of overlapping Access Points (APs) and inter-AP interference, researchers have focused on studying MAP-Co approaches for coordinated transmission in IEEE 802.11be, making MAP-Co a key feature of future WLANs. Additionally, the high overlapping AP densities in EHF bands, due to their smaller coverage, must be addressed in future standards beyond IEEE 802.11ay, specifically with respect to the challenges of implementing MAP-Co over 60GHz bands. In this talk, the state-of-the-art in MAP-Co features and their drawbacks concerning emerging WLAN, several novel future directions and open challenges will be provided.
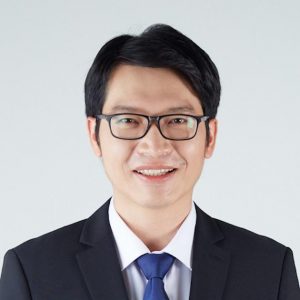
Prof. Joey Tianyi Zhou
A*STAR Centre for Frontier AI Research (CFAR) & the Centre for Advanced Technologies in Online Safety (CATOS), Singapore
Title: Dataset Distillation and Pruning: Streamlining Machine Learning Performance
Bio:
Joey Tianyi Zhou is a Principal Scientist, Deputy Director with A*STAR Centre for Frontier AI Research (CFAR), and he is also a Principal Scientist in the Centre for Advanced Technologies in Online Safety (CATOS), Singapore. Concurrently, is holding an adjunct faculty position at the National University of Singapore (NUS). Before working at CFAR, he was a senior research engineer with SONY US Research Center in San Jose, USA. Dr. Zhou received a Ph.D. degree in computer science from Nanyang Technological University (NTU), Singapore. His current interests mainly focus on improving the efficiency and robustness of machine learning algorithms. In these areas, he has published more than 100 papers and received the Best Student Paper Nomination at the European Conference on Computer Vision (ECCV’16), Best Paper Award at the International Joint Conference on Artificial Intelligence (IJCAI) workshops, and Best Poster Award and runner-up prize at International Conference on Computer Vision (ICCV19) on HANDS workshop and its competition, respectively.
Dr. Zhou regularly organizes workshops/tutorials at top-tier international conferences like CVPR, IJCAI, ICDCS, etc. He is serving as an Associate Editor and Editorial Board for Artificial Intelligence Journal (AIJ), IEEE Transactions on Emerging Topics in Computational Intelligence (TETCI) and IEEE Access, IET Image Processing, and Area Chairs in top machine learning conferences like ICLR, ICML, NeurIPS, IJCAI etc.
Abstract:
In the rapidly evolving field of machine learning, “Dataset Distillation and Pruning” has emerged as a key strategy for enhancing model efficiency. Dataset distillation involves extracting essential information from extensive datasets to create refined, smaller-scale data that maintains model robustness while reducing computational burden. It can be likened to distilling knowledge from vast amounts of data.
On the other hand, dataset pruning is akin to pruning unnecessary branches from a tree. This technique involves removing redundant or minimally impactful data points, resulting in a more streamlined, faster, and resource-efficient machine learning model. By eliminating extraneous information, dataset pruning aids in constructing lean algorithms with outstanding performance and without unnecessary computational overhead.
These two approaches collectively address the challenges posed by the abundance of data in the digital age. Dataset distillation and pruning complement each other in model compression research and further optimize the entire machine learning workflow’s energy consumption, ultimately facilitating sustainable deployment of large-scale data and models on endpoints.
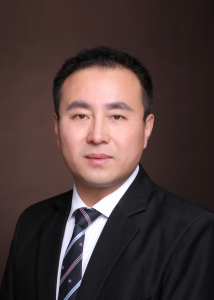
Prof. Guangjie Han
Hohai University, China
Title: Indoor Localization with Deep Gaussian Process and Uncertainty Maps
Bio:
Guangjie Han is a professor, currently serving as the Dean of the School of IoT Engineering at Hohai University. He is an IEEE Fellow, IET/IEE Fellow, and AAIA Fellow. His main research interests include smart oceans, industrial IoT, artificial intelligence, networks, and security. In recent years, he has published more than 350 high-level SCI journal papers, including over 130 papers in the IEEE/ACM Trans. series, in international journals such as IEEE JSAC, IEEE TMC, IEEE TPDS, and IEEE TCC. His publications have been cited over 17200 times on Google Scholar, with an H-index of 68. He has authored three monographs and translated one book. He has led more than 30 provincial and ministerial-level research projects, including national key R&D programs and national natural science foundation key projects. He has been granted 130 national invention patents and 6 PCT international authorized patents. He has received numerous awards, including the second prize of the China Business Federation Science and Technology Award, the third prize of the Jiangsu Provincial Science and Technology Award, the second prize of the Liaoning Provincial Science and Technology Progress Award, and the Best Paper Award of the IEEE Systems Journal in 2020. For five consecutive years (2019-2023), he has been listed as one of the top 2% of scientists globally, as well as for the Chinese Highly Cited Researchers list for four consecutive years (2020-2023). Currently, he serves as an associate editor for more than ten international journals, including IEEE TII, IEEE TVT, IEEE TCCN, and IEEE Systems. He has been awarded the “333 High-level Talents in Jiangsu Province” (second level), the “Outstanding Contribution Young and Middle-aged Experts in Jiangsu Province,” the “Minjiang Scholar Lecture Professor,” and the “May 1st Labor Medal” of Changzhou City.
Abstract:
The underwater acoustic sensor network (UASN) is the core module to realize the “smart ocean”. At present, the UASN has not yet fully played its role in the complex water environment. The fundamental reason lies in the lack of effective methods to ensure network security and reliable data transmission. This report mainly introduces the team’s research work on the trust management mechanism of UASNs. The main research contents include: 1) Intrusion detection algorithm based on energy consumption prediction model; 2) Multi-dimensional trust calculation algorithm based on fuzzy theory; 3) Trust evaluation algorithm based on cloud theory; 4) Trust cloud migration mechanism based on AUV; 5) Trust update mechanism based on reinforcement learning; 6) Anomaly-resilient trust model based on isolation forest. The research results have important theoretical value and practical significance for exploring the security technology and application of UASNs.